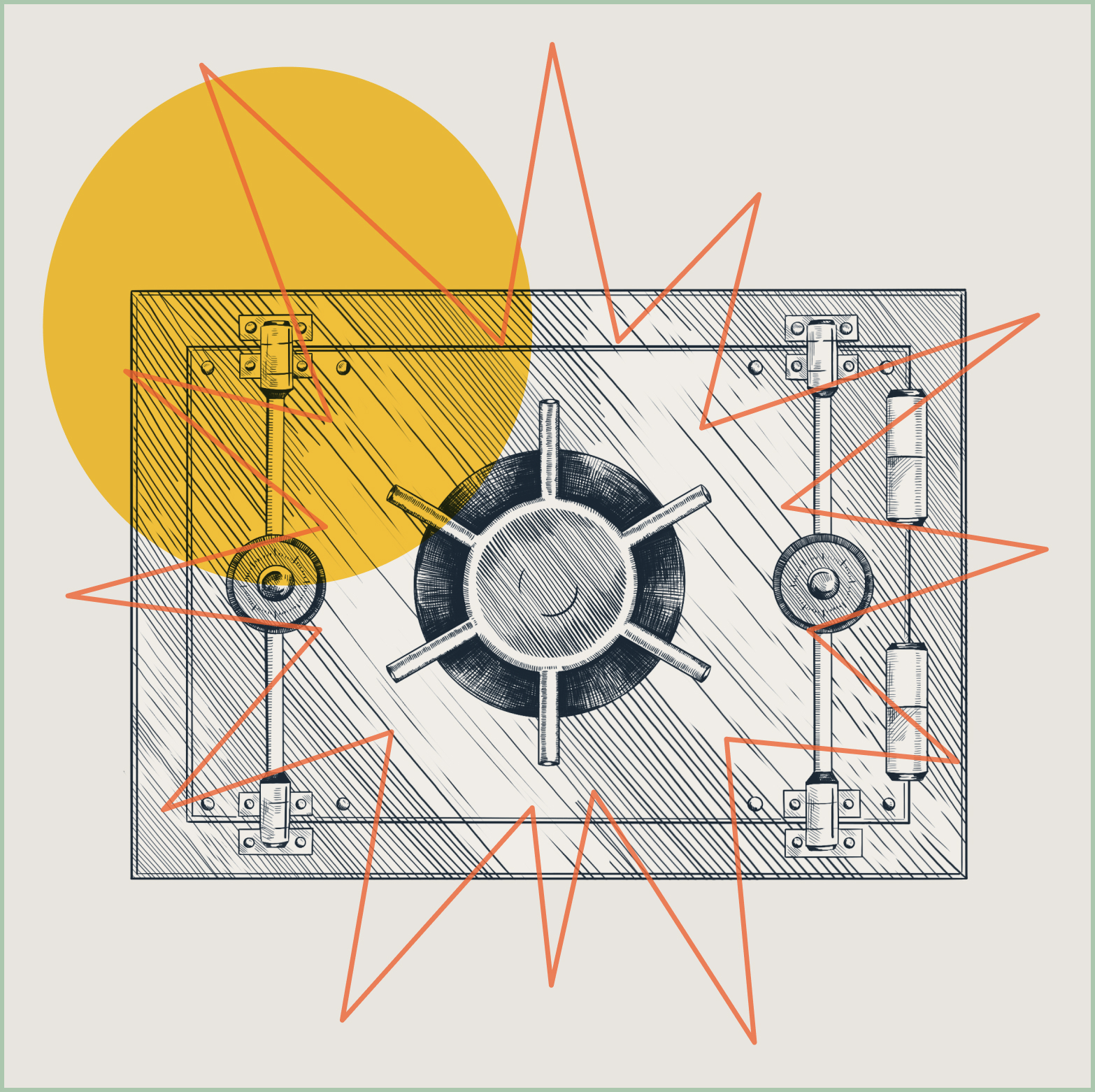
Automating the Grid: No Magic Button, Just Smart Tools
In my previous two-part blog series, we explored the fundamentals of Generation Interconnection (GI) and how the process plays a pivotal role in the clean energy transition. In Part 1, we looked at the GI process itself, highlighting the growing complexity of interconnection queues, the importance of injection studies, and the challenges around network upgrade costs and project withdrawals. In Part 2, we shifted the focus to software solutions, discussing how the right tools can help developers optimize their Points of Interconnection (POI), balance precision with scalability, and make smarter decisions in an ever-evolving GI landscape.
Fast forward to today, and the conversation has progressed. At the recent Federal Energy Regulatory Commission’s (FERC) Workshop on Generation Interconnection, industry leaders shared their insights on how automation and emerging technologies like AI can further streamline the GI process. In this post, we’ll explore key takeaways from the workshop, sharing how automation is set to transform the way we approach grid interconnection and why AI could be the next frontier in this space.
Automation: Great for Repetitive Tasks, Not for Solving the Universe
The conversation at the FERC workshop made one thing clear: automation isn’t a cure-all. It’s more like that super-efficient coworker who does all the boring stuff so the rest of us can focus on solving the real problems. Tasks like model building, data entry, and report generation are tedious but critical. Automating these allows engineers to free up their brainpower for bigger challenges like preventing grid congestion or planning future transmission networks.
One panelist noted that automation’s power lies in freeing up human capacity, rather than replacing it. Automation is the sidekick, not the superhero. By taking on the repetitive tasks, it lets engineers focus on more strategic thinking. This was particularly evident in discussions on system impact studies, where automation has shaved off significant time from the manual process of building models and running tests.
However, the challenges of automation were also highlighted:
- Standardization… or the Lack of It: GI procedures differ across regions like MISO, PJM, and SPP, with each having its own data formats, assumptions, and rules. It’s like everyone showing up to a potluck with a different recipe, but no one brought plates. For automation to reach its full potential, we need to standardize processes, or at the very least, ensure interoperability between different systems.
- Network Upgrade Cost Estimates: The Data Black Hole: Even with automation, developers still struggle to accurately estimate network upgrade costs because the information they need is often locked away by transmission owners. It’s like trying to fix a car without knowing what parts are broken. Greater data transparency here would be a game changer. For example, the impact studies for GI will highlight the part of the system that is congested but won’t tell us what exact equipment needs to be replaced to unlock the extra capacity, something that is key in accurate and reasonable network upgrade cost estimates.
- Automation and Non-Technical Tasks: Beyond technical studies, automation can also assist with administrative tasks like document review, site evaluations, and error detection. Think of AI and automation as the ultimate administrative assistants—helping to clear your inbox faster and keeping everything neat and organized.
AI: From Sci-Fi Dreams to Practical Grid Support
Much of the current hype around AI revolves around Large Language Models (LLMs), such as ChatGPT, which have shown impressive capabilities in natural language tasks. However, when it comes to AI, there’s both excitement and realism. As one panelist humorously noted, LLMs are great at writing essays and telling you what to say on your next Zoom call, but when it comes to structured data like power system models, they tend to trip over their own virtual feet. LLMs are amazing for natural language tasks, but they aren’t built for handling the nitty-gritty engineering data of a power grid.
However, AI’s potential in the GI process is still massive. Machine learning models trained specifically for energy systems could assist with:
- Grid Topology Conflict Detection: An AI that understands the grid like a mechanic knows engines could flag conflicts or mismatches in real time. Imagine how much faster projects could move forward with this kind of assistance.
- Study Auxiliary File Management: A well-trained AI model, familiar with the inputs required for grid reliability studies, could take on the tedious task of managing auxiliary files. For instance, when changes are made to a study model, the corresponding contingency files—which outline different grid conditions—must be updated to reflect the new grid topology. AI could automate this process, ensuring that auxiliary files are always in sync with the latest study model, saving engineers valuable time and reducing the risk of human error.
- Document Automation: Let’s not forget the unsung hero of automation—document generation. AI could speed up the legal review process, error-checking reports, and ensuring nothing gets missed in the piles of paperwork that come with every project.
Automation Won’t Replace Engineers, But It Will Need Their Help
One of the best points made at the workshop was that automation solutions can’t exist in silos. Power system engineers and software developers need to work hand-in-hand. Think of it like building a house: engineers lay the foundation, but software developers bring the tools that make construction faster, safer, and smarter. This collaboration is vital to ensure that automation tools are practical and solve real-world challenges.
Another consideration raised was that many RTOs and utilities don’t have the expertise or resources to build automation tools themselves. This presents a huge opportunity for solution providers to step in and offer scalable, flexible tools. Additionally, as automation reduces the reliance on human labor, we could start seeing constraints shift toward computing resources, leading to a new frontier of challenges to solve.
Finally, FERC could play a significant role by providing funding for these solution providers to innovate and drive the adoption of automation across the industry.
Conclusion: Automation Is Cool, but It’s No One-Size-Fits-All
While automation is clearly a key player in transforming the GI process, it’s important to remember that no single solution will work for every organization. Each utility, developer, and RTO operates with its own tech stack and workflows. For automation to truly unlock its full potential, it needs to be flexible and capable of integrating with different systems. The real magic happens when we combine customization with scalability.
The future is bright for AI and automation, especially when used to augment human decision-making. By working together, power system engineers and software developers can ensure that these tools are used to their full potential, helping us build a more efficient, resilient grid that’s ready for the demands of tomorrow.
Thanks again to FERC’s staff for organizing this insightful workshop and giving us a peek into what the future holds. The energy transition is happening fast—let’s make sure we’re moving with it, not against it.
Loved the article? Hated it? Didn’t even read it?
We’d love to hear from you.