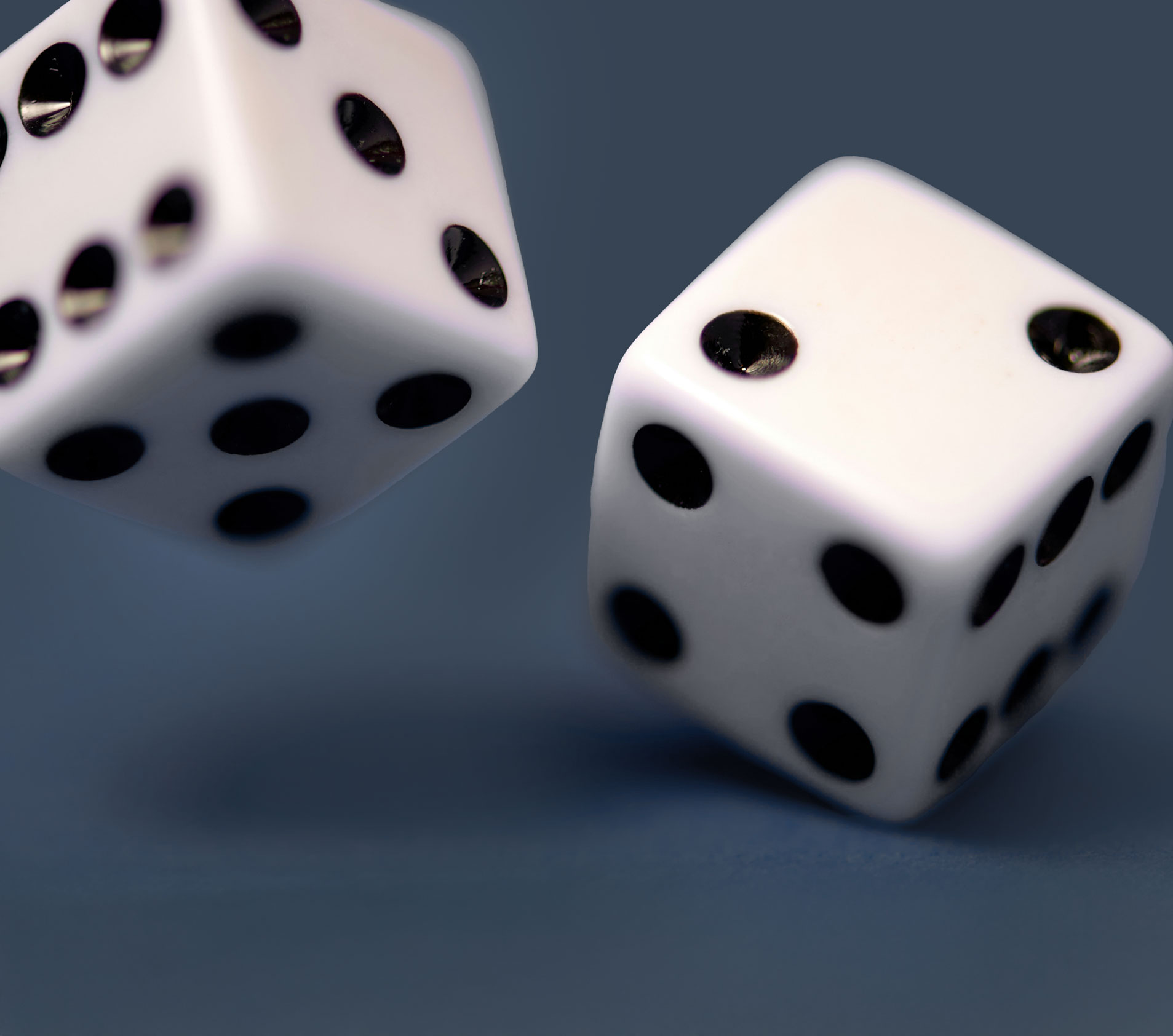
Addressing uncertainty in generation queues by using Monte Carlo simulations and machine learning to predict project withdrawals, offering a robust tool to manage cluster-based queue complexities.
Introduction
This poster presents a probabilistic framework for evaluating generation interconnection (GI) costs in cluster-based queues. Traditional GI assessments rely on fixed assumptions, but uncertainties—like project withdrawals—can significantly impact network upgrade needs. Our approach incorporates Monte Carlo simulations (MCS) with Definitive Planning Phase (DPP) data and long-term expansion models (MTEP) to capture a wider range of outcomes. Machine learning methods further enhance the analysis by estimating project withdrawal probabilities. The result is a more realistic, risk-informed assessment of GI costs, improving decision-making for developers and stakeholders.
Results
Deterministic vs. Probabilistic Cost Estimates:
- Deterministic results provide a single cost figure, but ignore the wide range of potential queue withdrawals and congestion outcomes.
- Probabilistic charts show a “band” of costs for different injection sizes, highlighting the likelihood of various upgrade scenarios.
Scenario Variability:
- In sample studies, scenarios with heavier congestion triggered upgrades at lower injection levels, raising overall costs.
- Scenarios with fewer overlapping GI projects showed a higher “clean injection capacity,” where fewer upgrades were required.
Actionable Insights:
- Developers can gauge how likely they are to incur certain upgrade costs based on different queue scenarios.
- Planners and policymakers gain a more holistic view of where to allocate resources for transmission improvements.
Methodology
Recommendation
Adopt Probabilistic Methods Early:
- Incorporate MCS in preliminary feasibility studies to better understand the “worst case” and “best case” cost outcomes.
Leverage Hybrid Models:
- Blend short-term GI queue data with long-term expansion plans to avoid underestimating or overestimating network congestion.
Explore ML Techniques:
- Use classification models to refine the probabilities of project withdrawal, increasing the fidelity of scenario analyses.
Encourage Stakeholder Collaboration:
- Transmission owners, regulators, and developers should jointly refine cost estimation guidelines and share data to improve the reliability of cost forecasts.
Conclusion
Our probabilistic framework addresses a key gap in GI studies by explicitly modeling the uncertainty surrounding cluster-based queues. Through MCS, stakeholders receive a fuller picture of possible upgrade requirements, ensuring that project evaluations are grounded in realistic probability distributions rather than fixed assumptions. By laying the groundwork for integrating machine learning, this approach is poised to evolve with richer data and predictive accuracy, aiding the industry’s shift toward more flexible, resilient, and cost-efficient interconnection processes.
Loved the article? Hated it? Didn’t even read it?
We’d love to hear from you.